Risk scoring with machine learning
A typical approach in estimating customers' credit risk is based on their repayment history and models such as logistic regression. This system, however, is limited to existing clients and tends to perform poorly on clients without a history of taking a loan.
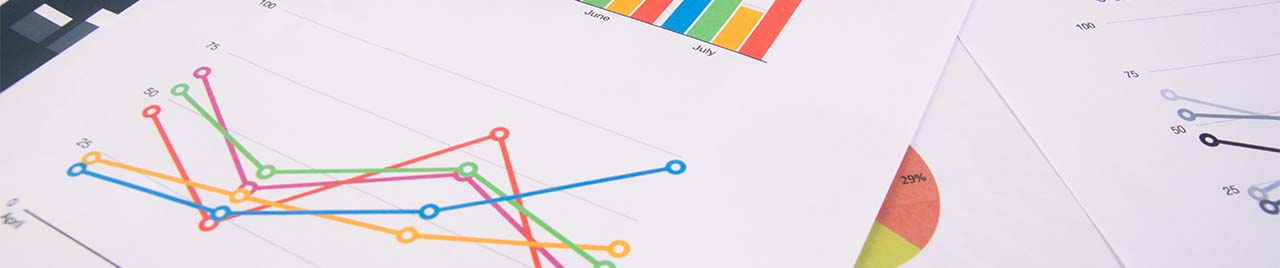
Business case
A significant improvement can be made by combining additional data sources with more advanced techniques, such as graph machine learning and natural language processing. These hurdles can be overcome by considering not only the client's loans and primary sociodemographic data, but also their digital trace, relationships with other entities, and the detailed structure of their bank transactions. This leads to better-performing risk models for existing clients and allows pre-scoring even for non-clients based on their behaviour online.
Solution
We use an ensemble of models, each focused on a particular angle of the customer's behaviour, and then combine them into a single unified model.
Just like in traditional credit risk scoring, we also include bank statements, transaction histories and statistics from application forms. We then dig deeper into the client's transactions and determine the purpose of each one of them, using methods based on natural language processing. This allows us to understand the customer's spending and earning patterns which help us get a more precise risk scoring.
We pair clients (and non-clients) with their identities in various digital sources (banking app, web browsing, loan calculator). That way, we understand the client's interests and concerns – both of which correlate with risk. After that, we link clients to each other based on mutual transaction histories, device usage, and other attributes. Graph Neural Networks then helps to transform these connections into a meaningful risk predictor for each client. Geo-location data are also included in the risk scoring model as an important predictor of the client's affluence. This is especially beneficial for non-clients where we typically don't have a transaction history.
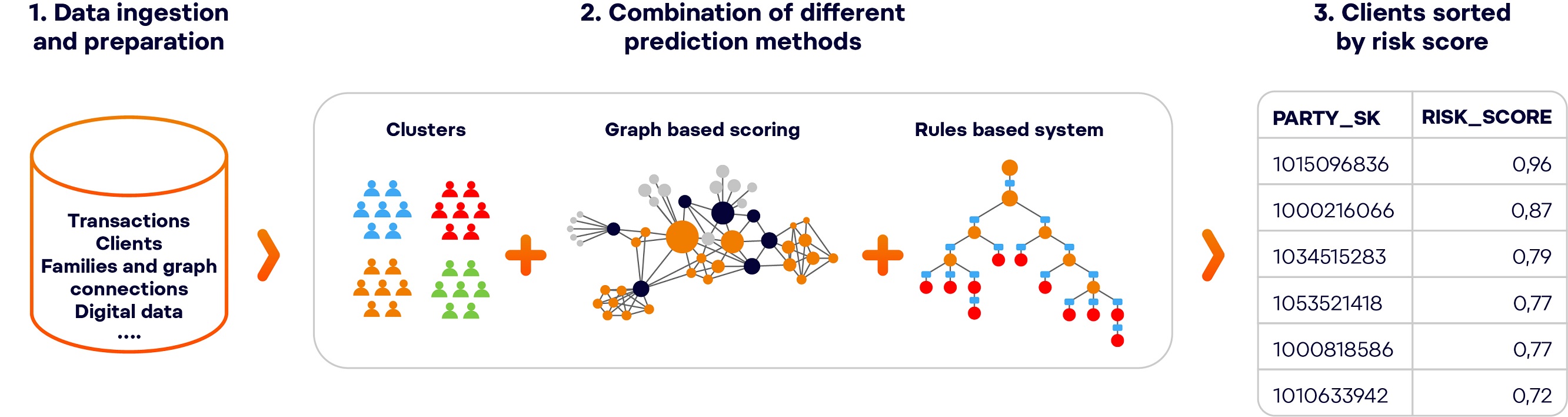
Benefits
- Natural language processing-based analysis enables credit risk scoring even for non-clients and provides an extensive risk profile for them.
- The precision of credit risk scoring can be highly enhanced in general for both clients and non-clients thanks to a focus on customer behaviour.
Enter your E-mail address and name to watch
Need to know More?
Ask us anything
Key contacts
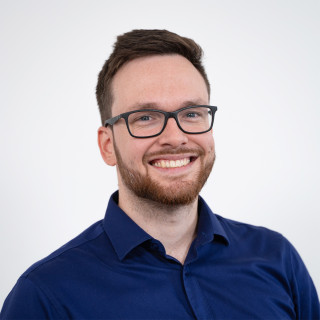
David Vopelka
FinTech lead | AI & personalisation expert