Fraud, Risk, and AML Detection
Enriching Core Fraud, Risk, and AML Detection Systems with New Data
Current fraud and anti-money laundering systems are too straightforward and not adapted to conditions of increased efficiency and might be ineffective due to high false-positive rates. They are based on expert rules, require manual adding and adjusting of scenarios and can not always detect implicit correlations.
The Challenges
- High false positive rates of standard rule-based solutions.
- Difficult to leverage new digital and unstructured data sources (e.g., interactions with online forms, suspicious inbound emails, ...).
- Maintaining compliance and keeping solutions up-to-date with new fraud patterns.
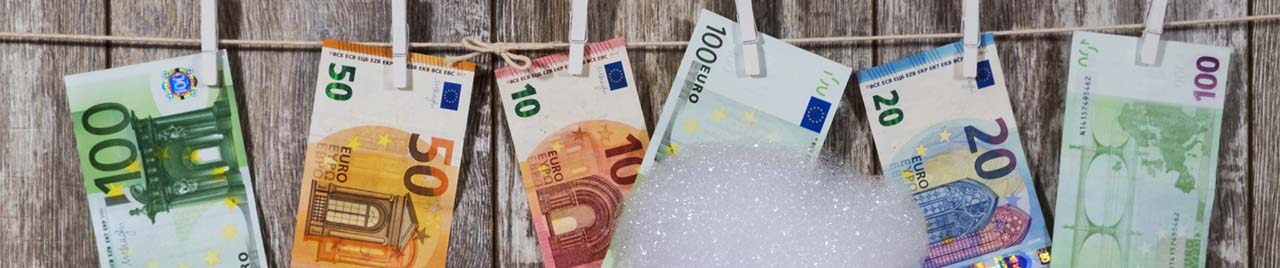
Our solution
AI solutions can help boost the security of digital transactions and online activities by analysing thousands of data points in real-time and flagging suspicious transactions or fraudulent claims before it leads to any loss for the company.
- AI/NLP approaches extract additional information about customers from new unstructured data sources, such as emails, calls, digital customer interactions, or complex customer relationships.
- End-to-end compliant machine learning, based on MLOps best practices, addresses the regulatory needs of fraud/risk solutions.
- Integration with your existing fraud management applications (SIRA, Netreveal, etc.) allows for reprioritization and the addition of new fraud cases.
- Automated interpretable risk scoring helps your specialists prioritize suspicious fraud/AML cases and investigate them faster.
Our solution is a tailored open platform to reduce false positives/alerts and boost the detection of true positives or actual frauds. Our platform enhances fraud detection by analyzing behavioral and unstructured digital data from various sources such as websites, apps, forms, and client connections. It also utilizes computer vision to score scanned documents. Additionally, advanced techniques like graph-based machine learning, clustering, and computer vision-based solutions, when combined with traditional rules, contribute to creating more robust solutions.
Read the Case Study:
Enhancing Fraud Detection with AI and Digital Data at Esure
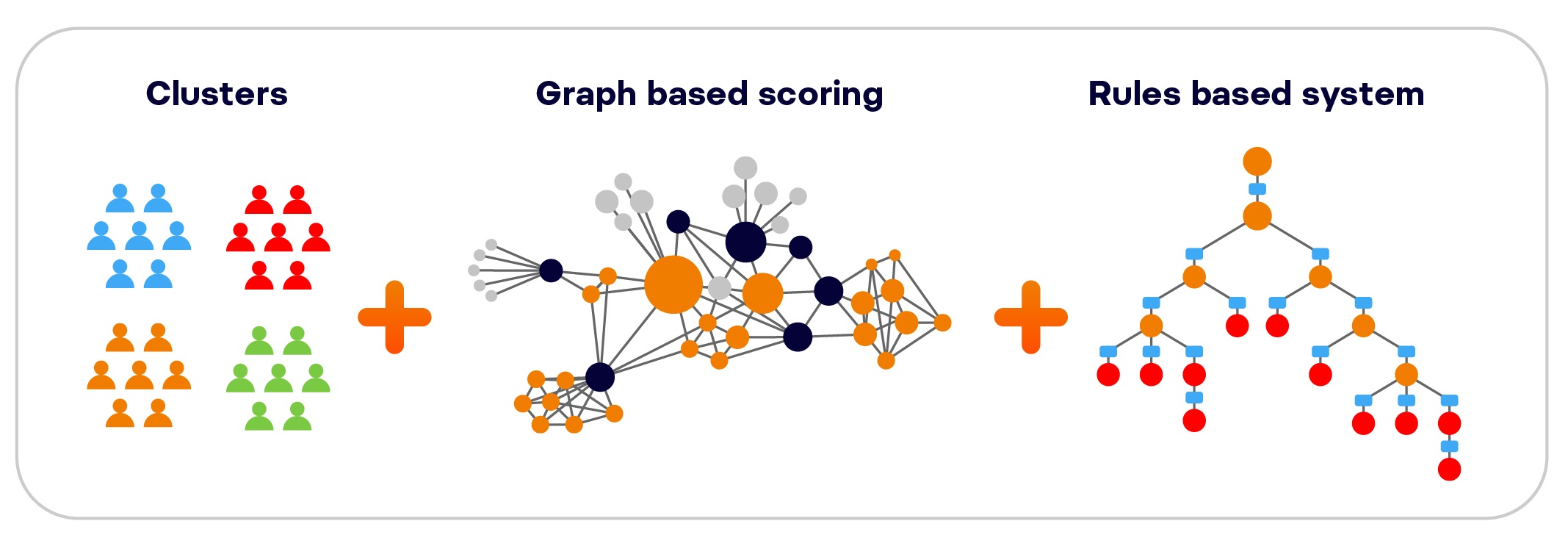
Benefits
- Significant cost savings by earlier fraud detection
- Detecting more fraud cases than previous rule-based solution and prioritizing them
- Saving time and bettering customer experience by lowering number of false positive alerts
- The platform is customized to your needs, providing explanations of clusters, classifying transactions, and prioritizing suspicious cases. This allows investigators to use their time much more efficiently.
Need to know More?
Ask us anything
Key contacts
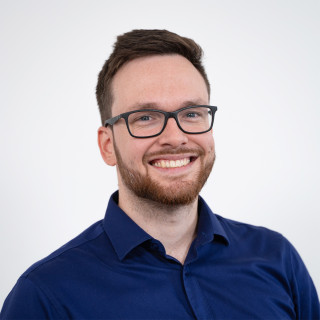
David Vopelka
FinTech lead | AI & personalisation expert