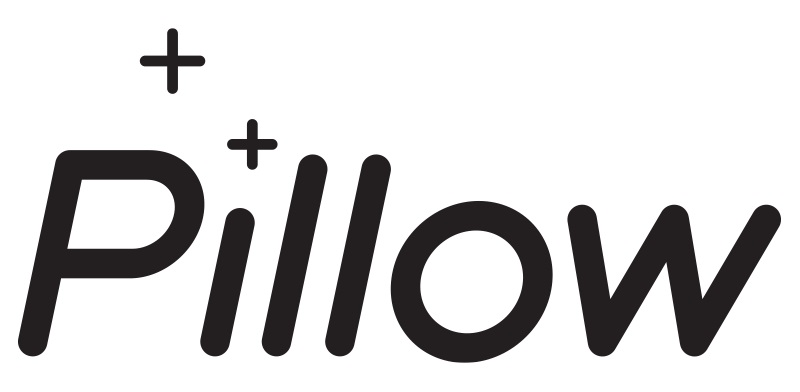
Profit increase
driven by utilizing predictions from the trained ML model and leveraging available data
Cost saving
achieved through streamlining and automating the entire process in Databricks and portfolio cleaning.
Easy to evaluate and extend results
to other Lines of Business by leveraging open-source technologies.
"DataSentics did excellent work at Pillow. They implemented a modern data platform Databricks and subsequently worked on a use case involving personalized pricing in non-life insurance. They quickly understood our business needs and delivered a functional solution. They also upskilled our internal team, enabling them to continue working independently with Databricks in the future."
The Challenge
Personalized pricing in the competitive motor insurance market
Given the dynamic and highly competitive nature of the motor insurance market, adopting flexible and personalized pricing strategies has become essential for success. The ability to quickly adapt to market changes and offer tailored pricing is now a critical differentiator in this industry. The renewal process for motor insurance was suboptimal and outdated. It heavily relied on semi-manual methods that incorporated inflexible spreadsheet rules. Consequently, this approach was labor-intensive, making it time-consuming and challenging to adjust as needed. Additionally, it was difficult to evaluate and optimize due to the rigidity of the methods used. Another significant drawback was that the process failed to utilize all available data, which could have otherwise enhanced decision-making and pricing accuracy.
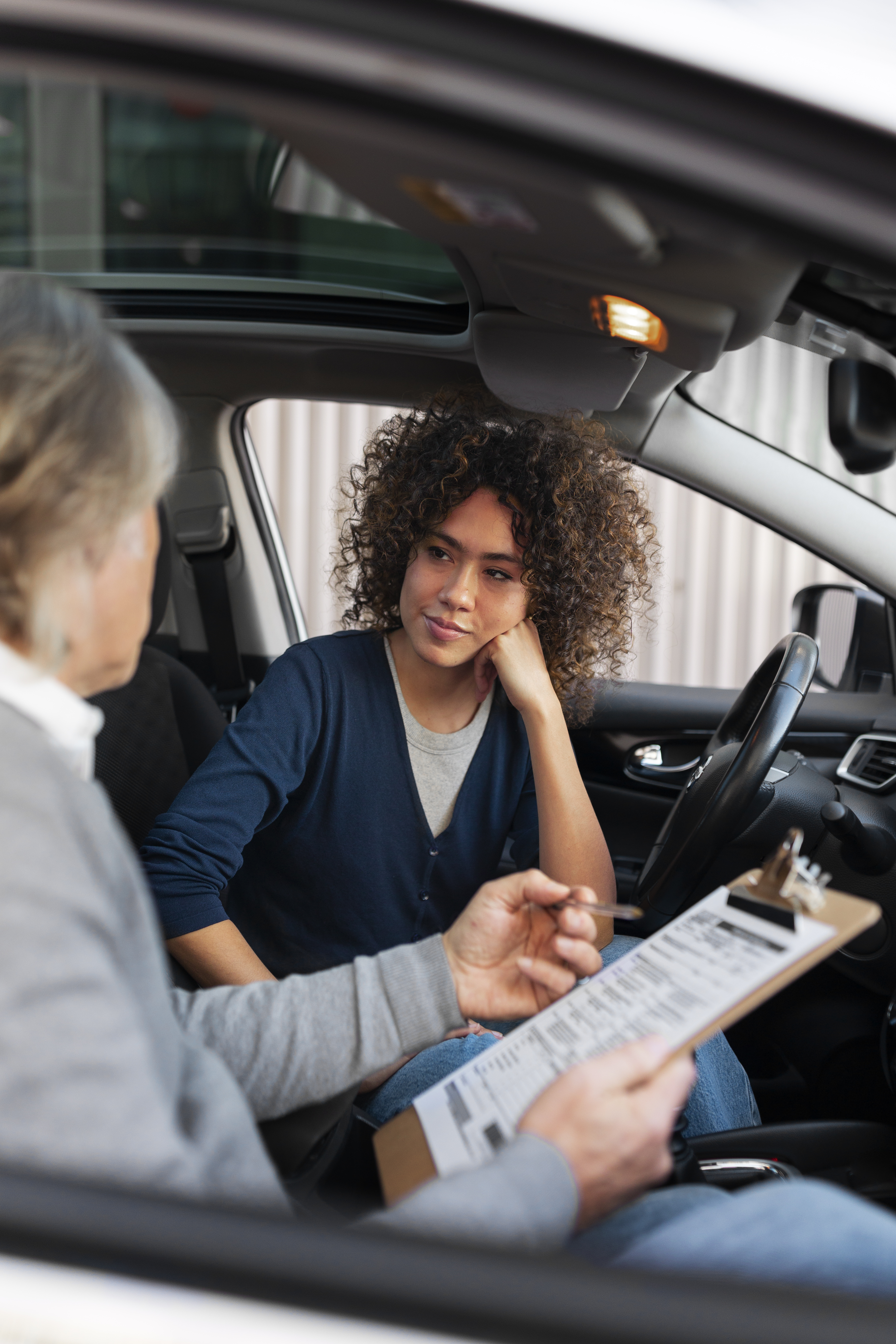
The Solution
Utilizing predictions from the trained ML model and leveraging available data
We used Azure Databricks to manage the entire pricing process, including data ingestion, churn modeling, optimization, and data publication. We developed a robust data ingestion pipeline that integrates all available data sources and trained a churn model using advanced ML methods like XGBoost. This model determines the optimal renewal price for each customer, maximizing profit while aligning with our business strategy.
To ensure transparency and understanding of the model results, we integrated a detailed evaluation dashboard within MLFlow. This dashboard provides continuous insights into the model's performance. Additionally, we implemented rigorous checks to ensure consistency and reliability in our processes. By leveraging Azure Databricks and modern machine learning techniques, we have significantly improved the pricing strategy and increased profitability.
The Benefits
Increasing profit while maintaining the same churn rate
The new renewal process, fully based in Databricks, is now usable for the entire motor insurance portfolio at Pillow, meaning it is applicable for all vehicle types. By utilizing the outcomes from the trained ML model, the client can now better predict the price elasticity of their customers and react accordingly during the renewal process. Additionally, this process enables the client to more effectively detect risky clients and take this information into account during renewals.
Another benefit of the new solution is the potential rollout to other lines of business. Thanks to the use of open-source technologies, this expansion aims to be easy and cost-effective.