AI tech dilemma: Black Box vs Home-made, and can you have the best of both?
Artificial intelligence and machine learning are quickly becoming a part of our day-to-day lives. Many companies use advanced solutions to improve their efficiency in customer service or marketing, explore new business opportunities, or gain an advantage over their competition. However, modern, data-driven solutions also involve a big investment and require very careful planning and consideration of many factors, such as cost, timeframe, or skilled employees. And that’s where arises the crucial dilemma: Build or buy?
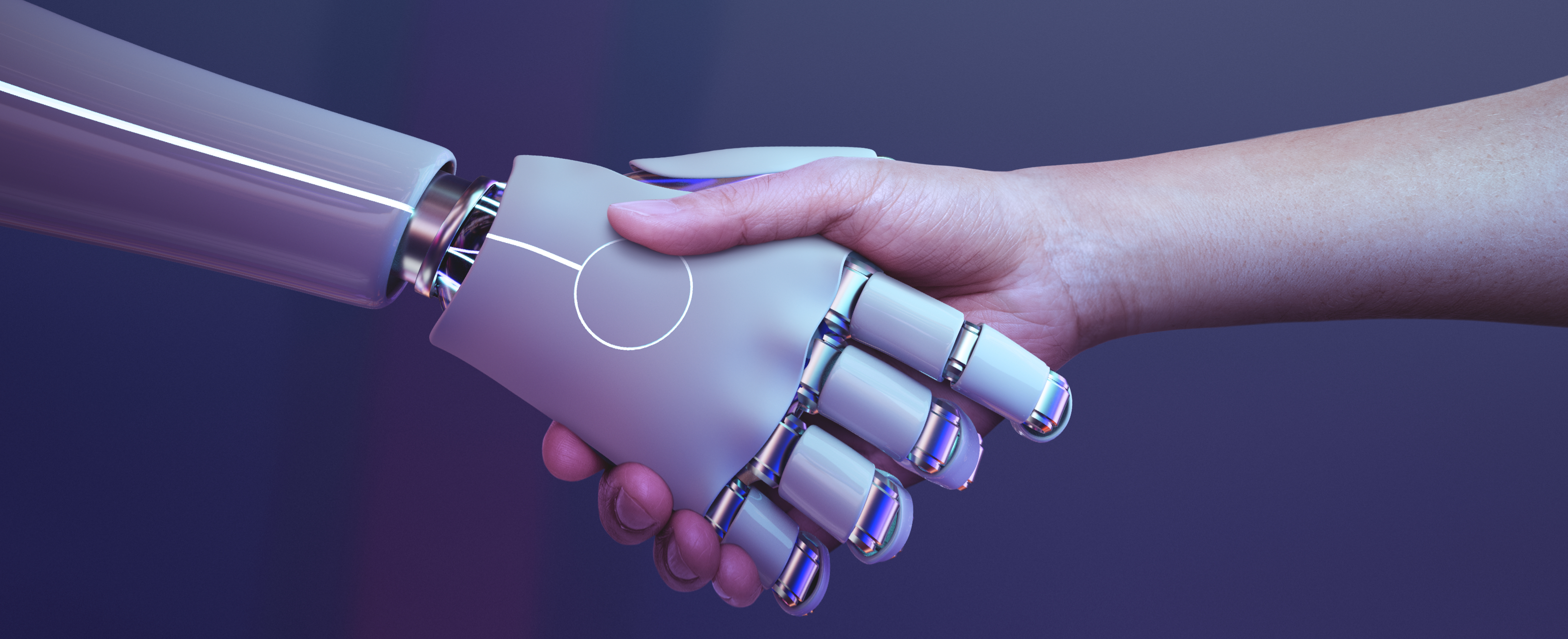
How important is it to know how a machine thinks?
The question isn’t “to adopt or not to adopt” the AI/ML solutions anymore. It’s how to adopt them. According to Forbes, 90.9% of firms identify the people and process challenges as the biggest barriers to becoming data-driven organisations when building their own AI solutions. Buying an off-the-shelf (also known as AI on tap, AI as a Service and productised AI) black box solution is the easiest and most accessible option. You’ll be buying a ready-made product with professional support and maintenance, but on the other hand, you won’t have full control over the data and might have to pay for features that you will not use. In this situation, fine-tuning and optimising the algorithms to get the best possible performance for your company may be an issue as you only can change and adjust what the vendor allows you to. Another issue, and likely the biggest one, is transparency.
Facing similar issues? Let's get in touch!
The black box allows you to see the input and output, but none of the processes and decisions that happen in between. This is not necessarily wrong. The neural networks in the black box AI can be so complex that humans are not able to explain nor understand the why and how of these outcomes, even when they prove to be accurate. There are situations when you do not really need to see what’s going on inside, as long as you can see that the program works the way it is supposed to. But this approach can be problematic and even dangerous in certain fields.
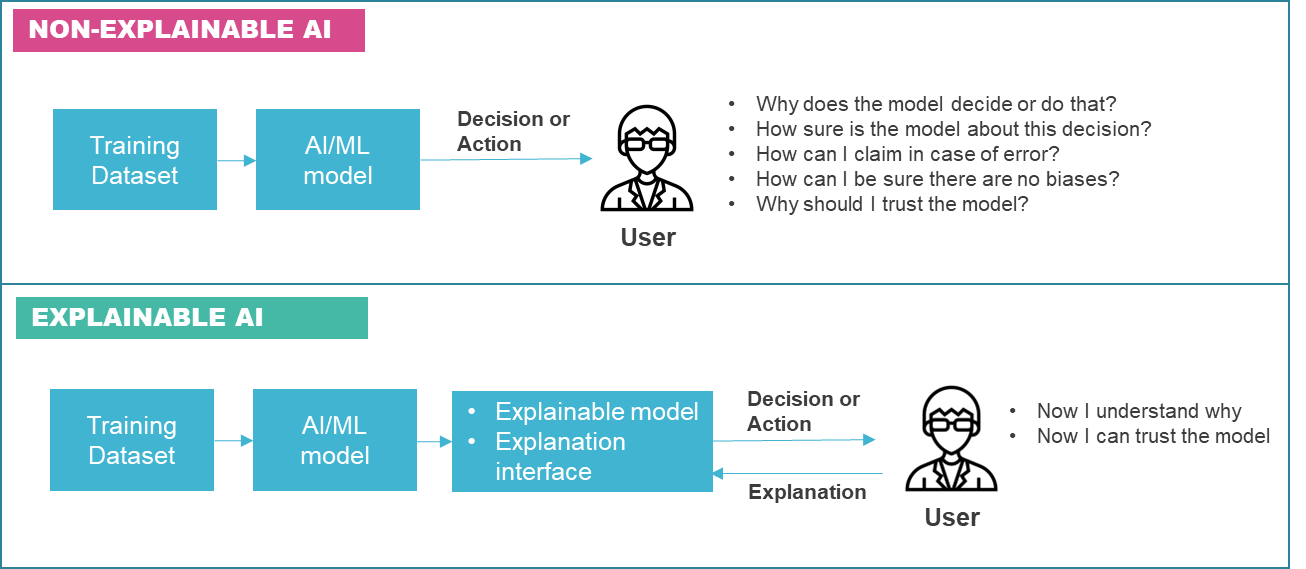
Source: Worldline
In 2018, during the wait for the Explainable Machine Learning Challenge winners announcement, the audience, consisting of experts in the fields of finance, robotics, and machine learning, were asked to answer the following question:
Suppose you had cancer and needed surgery to remove a tumour. Would you choose a human surgeon with a 15% chance of death during the surgery or a robotic arm with only a 2% chance of failure?
The answer seems obvious, and the majority of the audience did vote for the robot. However, there’s a catch! You must trust the robotic arm completely – there are no questions and no explanation of its decisions. These two options were supposed to simulate the difference between black box and white box AI and ask another question: Why must the robot be a black box?

The lack of explanation of the black box’s decisions sparked debates, and many people have become suspicious of it. The issue is that when you don’t see into the decision-making process, for example, when the AI is in charge of approving loans, it is very difficult to detect wrong decisions based on biased data or inadequate modelling techniques. Consequently, this can put you in a very uncomfortable position - especially when these decisions affect people who trust or depend on your company.
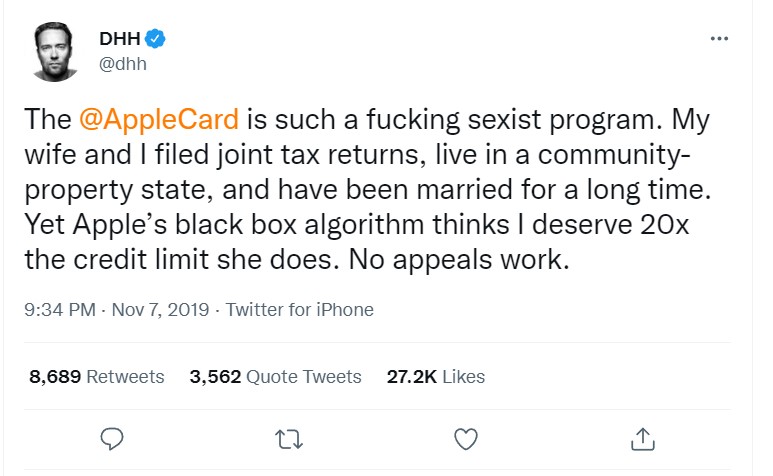
Is there a way to avoid pre-built black boxes and have everything under control? Yes! However…
AI, machine learning and data science have become a necessity for many businesses, both small and big. Countless advancements of these modern technologies, such as language processing or pattern recognition, will fuel the future of each business. And while using off-the-shelf AI solutions has its undeniable advantages, many companies prefer to build their own solutions tailored for their specific needs.
If you decide to build your own AI model workflow, you will have full ownership and control over the algorithm, but you must do everything from scratch. AI models built in-house are incredibly time and money consuming long before they even begin to be useful – we’re talking months or even years. Since AI technologies are changing and progressing so quickly, you also need to remember the constant need for maintenance, including never-ending research and development. Also, remember that creating a good model from the data science point of view is only one part of the job. Putting the model into the production pipeline, connecting it to other systems and automating the data ingestion and output may be a very challenging task requiring different sets of skills.
Another point to consider is that according to a prediction by Gartner, 85% of AI projects will fail due to the data, algorithms or the teams responsible for managing them. The bottom line is that you need to think through very carefully what you need, what is available to you, and what you can do before making the decision on how you want to adopt your AI solutions.
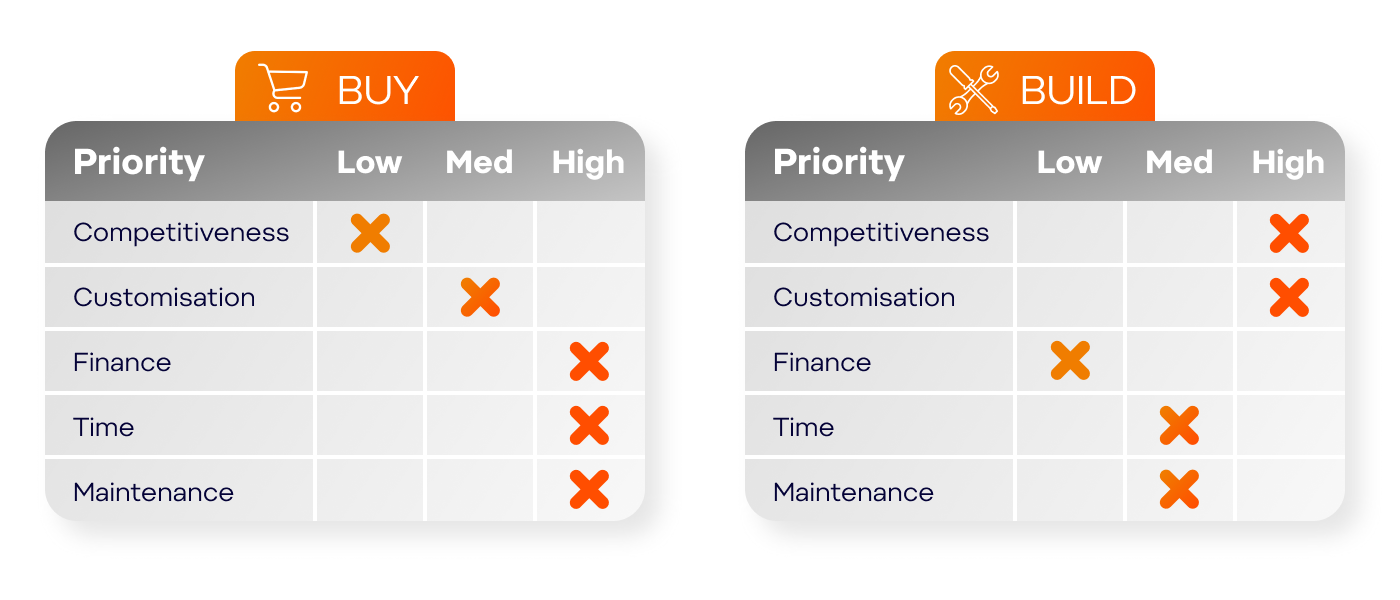
What if maintenance and availability didn’t have to be sacrificed for transparency and customisation?
The third option after home-made and off-the-shelf black box AI is a white box. The key difference between black box and white box AI is the transparency of the why and how behind the conclusions. The white box is much more interpretable than the black box - meaning the user can see into the process of decision making. That’s why these are preferred in core business functions and processing personal information.
Until quite recently, this level of transparency was only required in certain industries, such as the financial services. However, more and more attention is now paid to how personal data is used and that results in new requirements for accountability of AI/ML models. And, last but certainly not least, a crucial benefit of an interpretable white box model with transparent predictions is the customisation. Having the decisions and features under control is a big step towards better understanding and hence leveraging the possibilities of artificial intelligence for your business.
At DataSentics, we want to give you the best of all of these options. Our delivery model offers the convenience of SaaS and the level of control and ownership of custom-made software, making us not a regular service provider but rather a strategic partner.
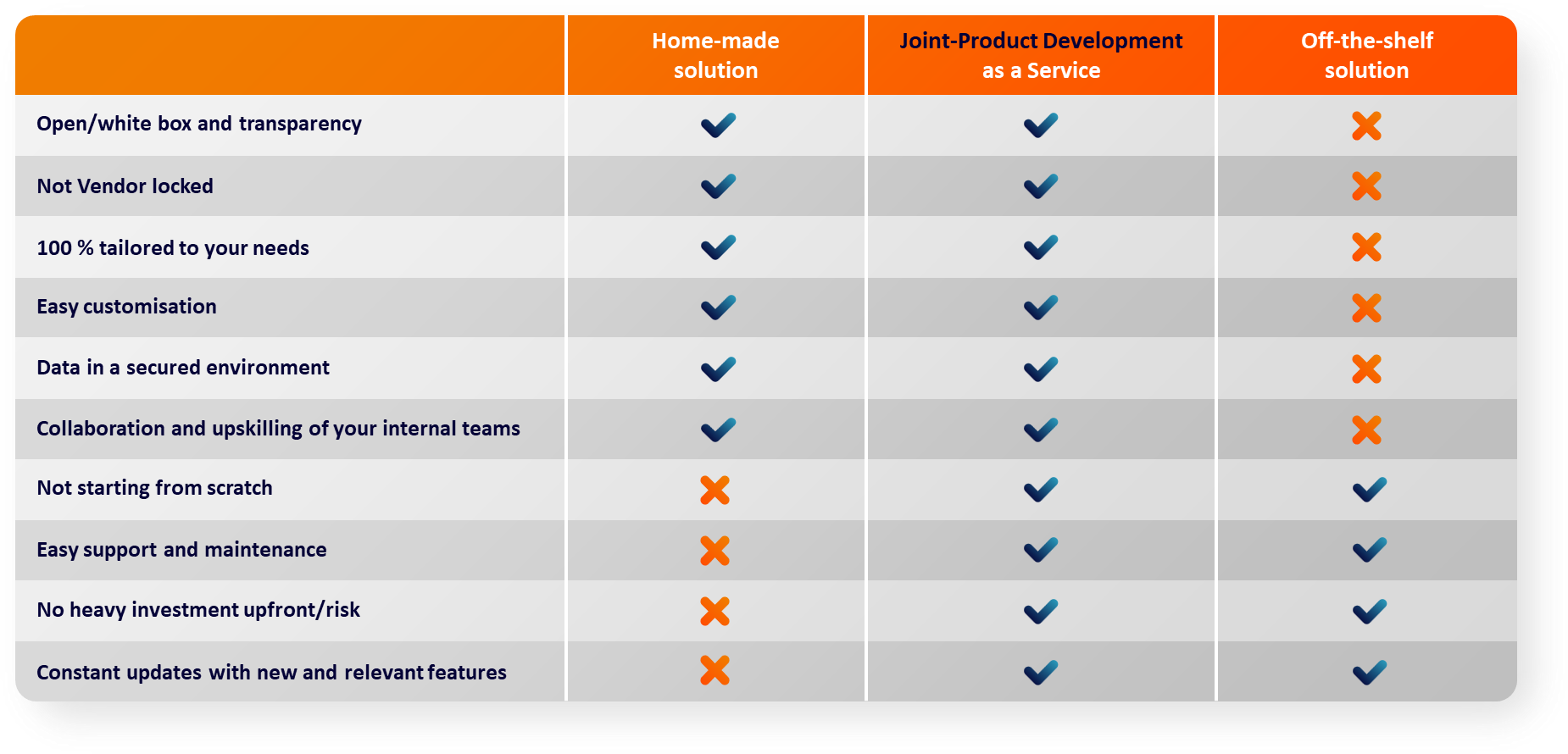